Trusted by job boards, HR platforms, ATS, and recruiters
The Most Advanced and Accurate AI Resume Parser
Steps to use our resume parsing software
Drag and drop a resume file or click to upload
PDF, doc, docx or word file (maximum 5 files)
Trusted by job boards, HR platforms, ATS, and recruiters
The Most Advanced and Accurate AI Resume Parser
Steps to use our resume parsing software
Drag and drop a resume file or click to upload
PDF, doc, docx or word file (maximum 5 files)
How Our Resume Parser Algorithm Works?
Our AI resume parser algorithm works in 4 steps
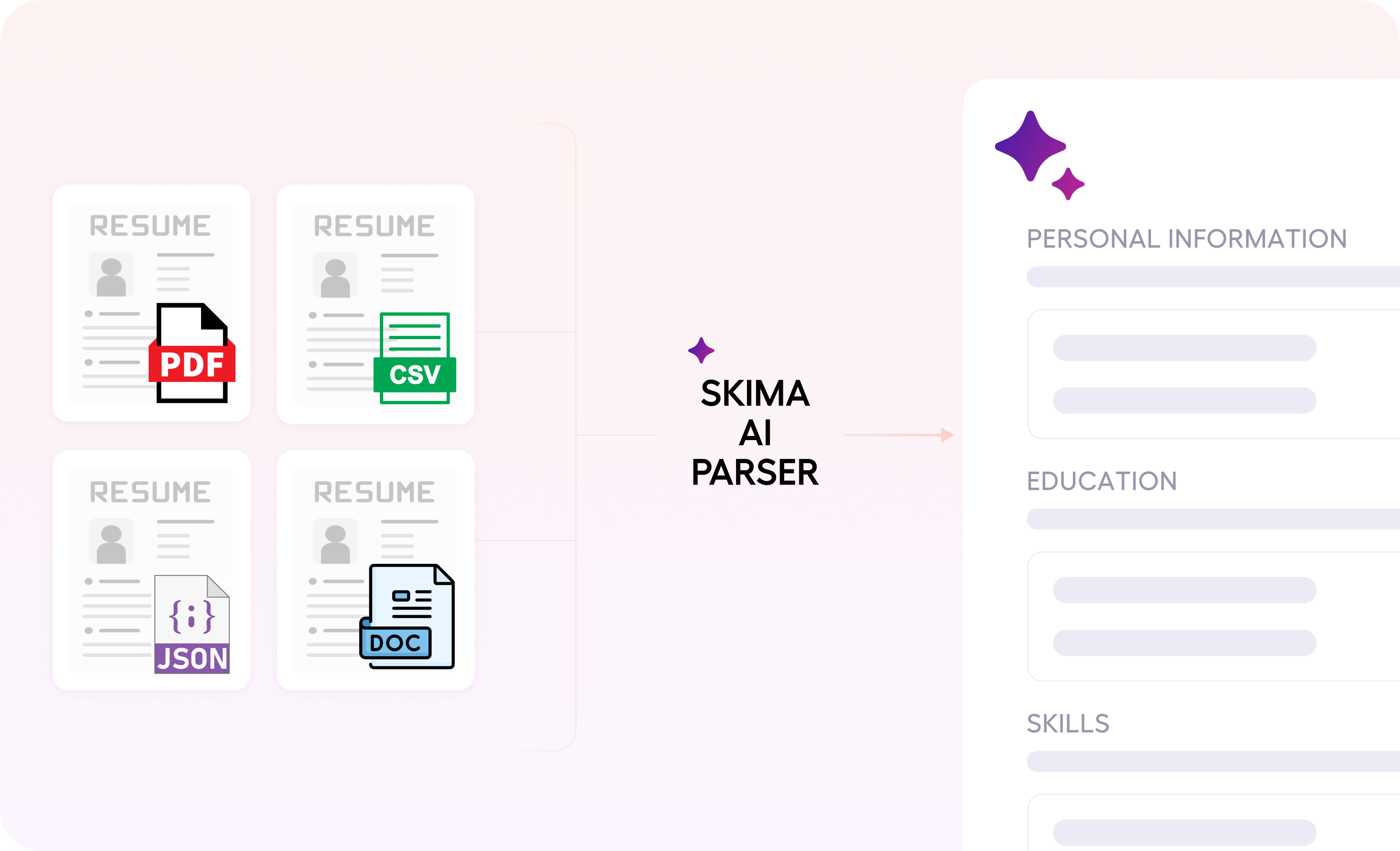
Step 1 - Extract Text from Any Document Format
Our resume parser algorithm first identifies and extracts raw text from formats like PDF, Word, and HTML. It uses OCR for image-based files and ensures text accuracy, which is crucial for ATS friendly parsing.
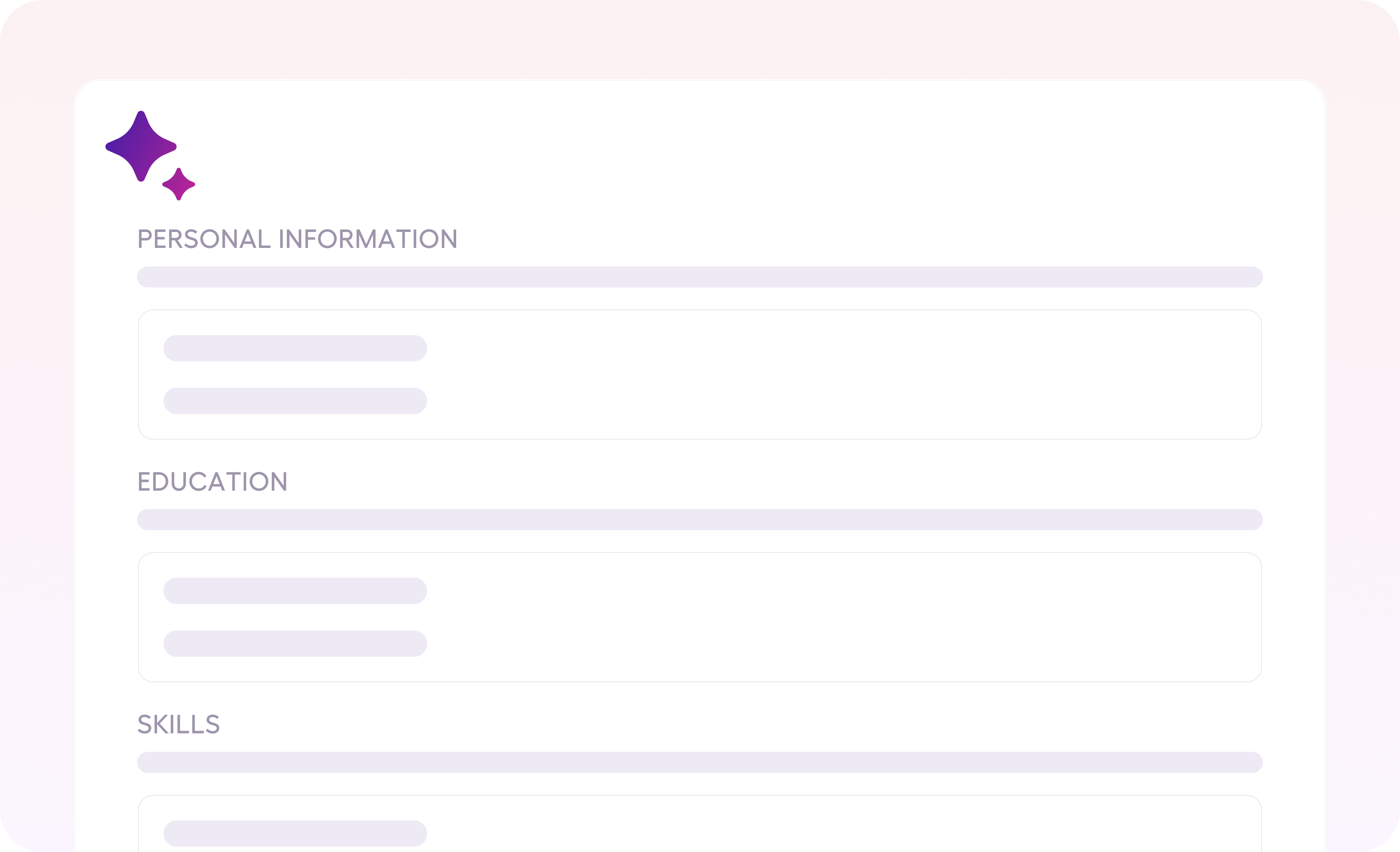
Step 2 - Organize Data into Logical Sections
The extracted text is then organized into sections like work experience, education, skills, and more. The parser's advanced pattern recognition ensures structured segmentation even in varied resume layouts.
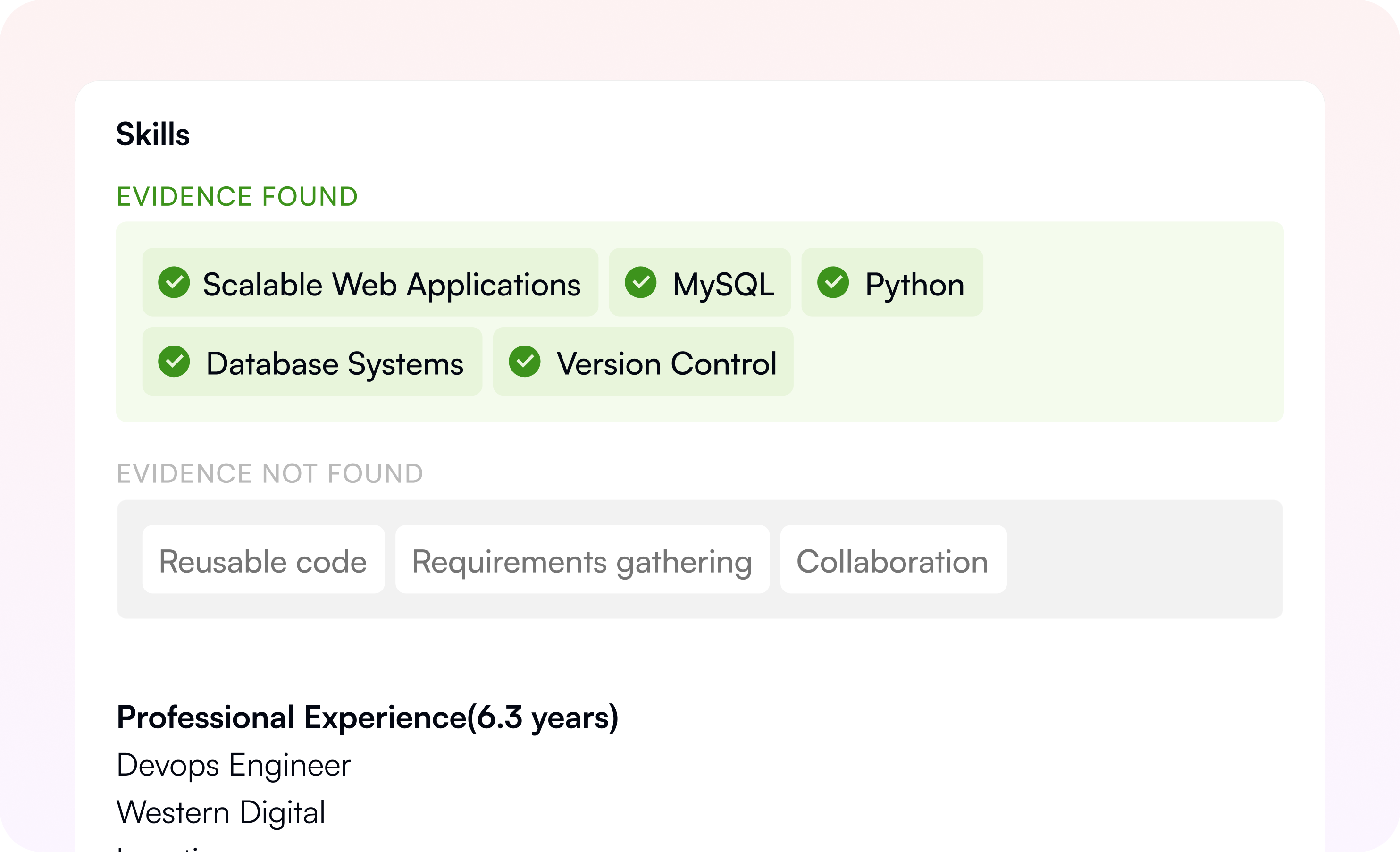
Step 3 - Prioritize Relevant Information Using NLP
Natural Language Processing (NLP) identifies and ranks essential details, filtering keywords and phrases that match job requirements. This prioritization optimizes candidate relevance for recruiters.
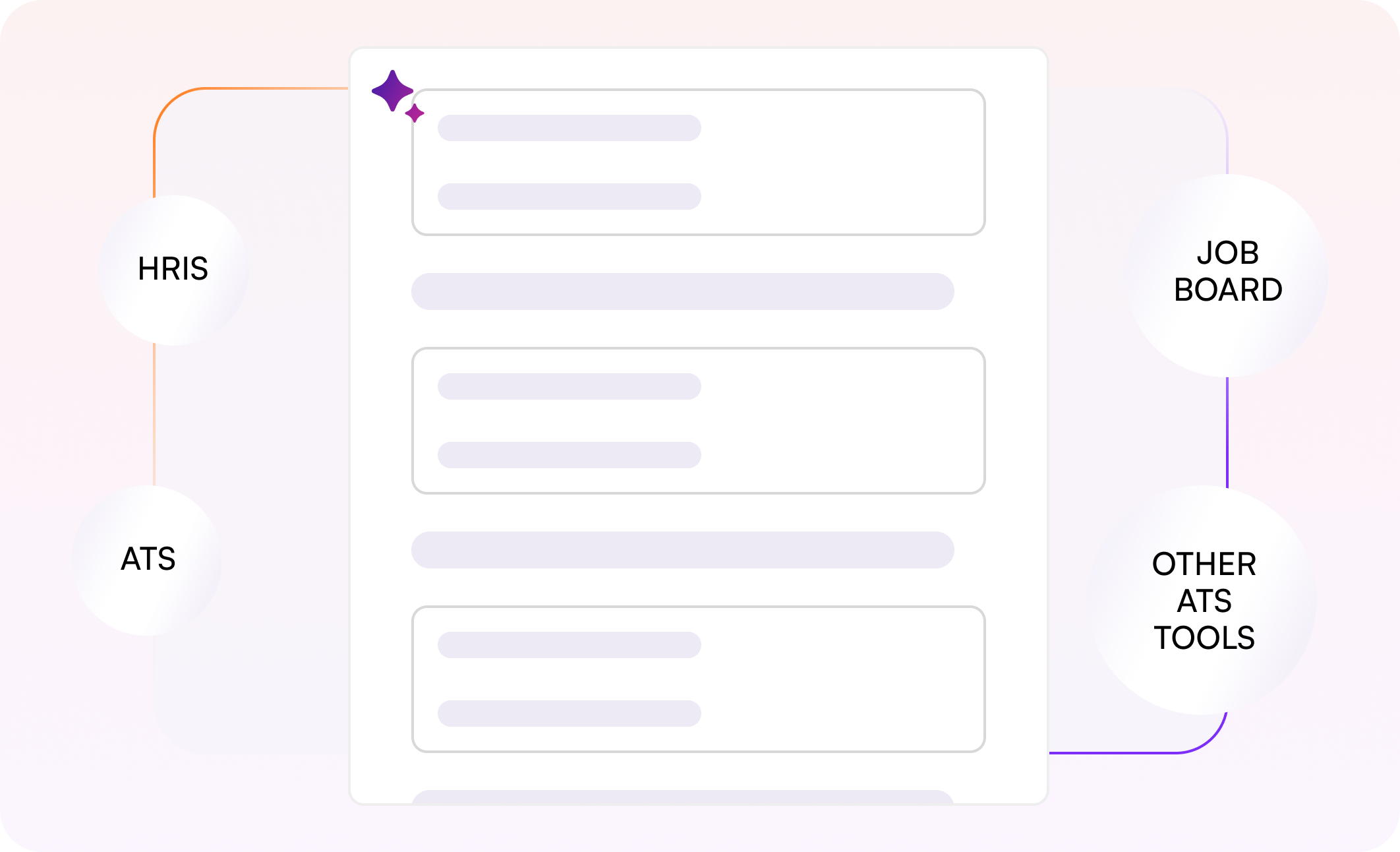
Step 4 - Output Optimized for ATS Systems
The final structured data is formatted to be ATS-compatible, ensuring easy integration with HR software. This step maximizes candidate visibility and accuracy in ATS filtering.
Why Choose Skima Resume Parser
Our Resume Parser Stand Out
High Accuracy at Speed
Our resume parser extracts 200+ key data points in seconds. You can parse 100,000+ resumes in minutes with unmatched speed and accuracy. Our AI-powered Resume Parser API simplifies resume data.
Multiple Formats
You can parse resumes in PDF, DOC, DOCX, IMG, TXT, HTML or Word format and export them as CSV, JSON, XLS, DOC, or PDF. You can configure our AI Resume Parser API to customize resume export templates.
Integrate your ATS
Our resume parser API integrates seamlessly with your HRIS, ATS, and other HR tools. This enables a smooth and automated data flow. Our CV parser helps you hire strategically with accurate and efficient results.
Skima AI vs. Other Platforms - The Competitive Edge
How does Skima Resume Parser stack up against other recruiting solutions like LinkedIn Recruiter, hireEZ, or SeekOut? Here are key differentiators that make Skima the smarter choice
Feature | | Other Platforms |
---|---|---|
Accuracy | ~99% accuracy, supports multiple formats | 70-88% accuracy, limited formats |
Format Support | Doc, Docx, PDF, Word, IMG, HTML, TXT | Primarily supports PDF, while few others also supports Doc, Docx, HTML, TXT |
API Integration Ease | Yes | Yes |
Cost Efficiency | Yes | No |
Customer Support | 24/7 | Mostly Email and Chatbot based support |
Data Security | SOC 2 and GDPR compliant | Not Complaint |
When it comes to resume parsing and candidate matching, Skima AI outperforms traditional competitors by combining advanced AI-driven accuracy, faster processing, and unmatched security. Unlike legacy systems that rely on basic keyword matching, Skima understands context, extracts key insights, and ranks candidates intelligently—helping recruiters make faster, data-driven hiring decisions. Skima Resume Parser has shown proven ROI improvements, reduced time-to-hire, and seamless integration
Trusted by Leaders
Recruiters and HR leaders are already experiencing the Skima AI advantage. Here’s what some of them have to say about how Skima transformed their hiring
“Skima.ai has drastically reduced the time we spend screening candidates by providing highly accurate AI-driven matches.” The quality of candidates we get is outstanding – no more endless resume skimming
– Jordyn Franci, Recruitment Head
“It completely revolutionized the way we hire.” Skima offers an all-inclusive set of AI-driven tools that pinpoint top contenders instantly. The AI Matching Score gives us an instant read on the best candidates, and the fast AI search helps us fill positions with minimal delay. We’re hiring faster and smarter than ever.
– Hiring Manager, Fortune 500 Company
“Better matches, faster hires.” We needed to fill niche roles quickly, and Skima delivered. Using Skima, Our Smarter Workforce team cut down time-to-fill by finding the right candidates faster. It’s like having a supercharged recruiting team working 24/7.
– Surendra T, Talent Acquisition Manager
Skima has completely transformed our sourcing process. Instead of spending hours manually searching, we simply ask the AI assistant what we need, and it delivers highly relevant candidates in seconds. It’s like having a recruiting co-pilot that taps into a vast global talent pool beyond our database. Absolutely game-changing!
– Animesh B, Sr. Recruiter
Many organizations – from high-growth startups to large enterprises – have joined the Skima AI revolution. 85% of recruiters who use Skima witness a double-digit improvement in hiring efficiency within months, and teams report a 5X faster candidate screening process. The success stories keep rolling in, as Skima AI consistently helps companies hire better talent, faster.
Get Started – Join the Future of Recruiting
Ready to transform your talent acquisition strategy? Don’t get left behind in the old way of recruiting. Embrace the power of AI and join the future of recruiting today with Skima AI.
Frequently asked questions
Everything you need to know about the product
Resume parsing is an AI-driven process of extracting information from resumes or CVs. The information includes education, experience, skills, contact details, and more. After extraction, the resume parser quickly turns unstructured data into organized fields. This process speeds up candidate filtering by up to 70% and improves hiring efficiency.
To parse a resume, upload it to a resume parser tool or ATS with parsing capabilities. The tool scans the document, extracts relevant information, and converts it into a structured format. Now, you can download or sync with your ATS, HRM, and other HR tools to easily search and filter.
Resume parsing uses machine learning and natural language processing to analyze resume text. It identifies patterns to classify data like names, skills, job titles, education, and more. The parsing process takes unstructured information from resumes and organizes it into structured fields. This organized data is then stored in the recruiter's ATS to filter and search for candidates by relevant qualifications.
Recruiters can save more than 70% of their time by using a resume parser tool. It speeds up recruitment by automating data extraction, reducing manual entry, and enabling recruiters to search for specific skills or experience. This technology boosts candidate searchability and ATS accuracy. This helps recruiters find qualified candidates faster and hire them sooner.
Yes, your data is extremely secure. Skima AI resume parser is SOC 2 compliant and follows strict security standards. It uses end-to-end encryption and secure storage. This setup protects sensitive candidate information from unauthorized access. So, both recruiters and applicants can be confident in their data privacy.